The challange
preventing downtime and related losses
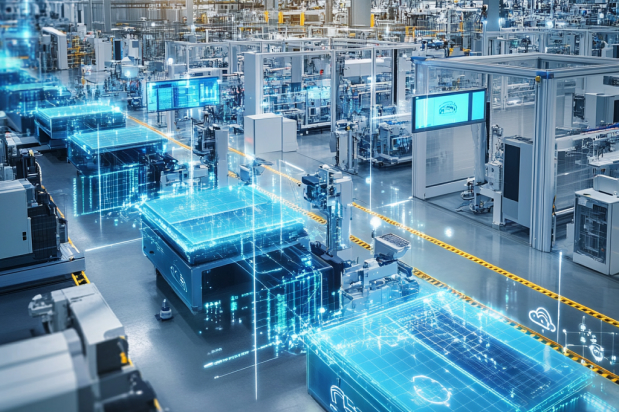
Manufacturing companies often experience sudden downtime due to unexpected machinery failures. Every hour when production stops can lead to delays, wasted resources, and significant revenue loss—often in millions of pounds.
Traditionally, maintenance followed a reactive approach—fixing when broken—or a preventive schedule—service at fixed intervals. However, both these approaches pose tangible problems to manufacturers.
With production machinery becoming increasingly complex, companies need smarter, more data-driven equipment monitoring solutions—and the advent of IoT makes it possible.
Reviewing available solutions
In the past, manufacturers typically relied on one of these approaches:
#1: Reactive maintenance
As easy as it sounds, reactive maintenance often results in prolonged downtimes as repairs happen after a failure. This unpredictability significantly impacts production schedules and drives costs up.
#2: Scheduled preventive maintenance
Although more secure than reactive methods, preventive methods involve servicing machines at fixed intervals, regardless of their condition. This often leads to higher maintenance costs and resource waste.
#3: Manual inspections and diagnostics
Manual checks of machinery conditions are manageable in small production setups, but they become a burden when companies scale production lines. They are labour-intensive, prone to errors, and fail to deliver the reliability and cost-efficiency modern manufacturers need.
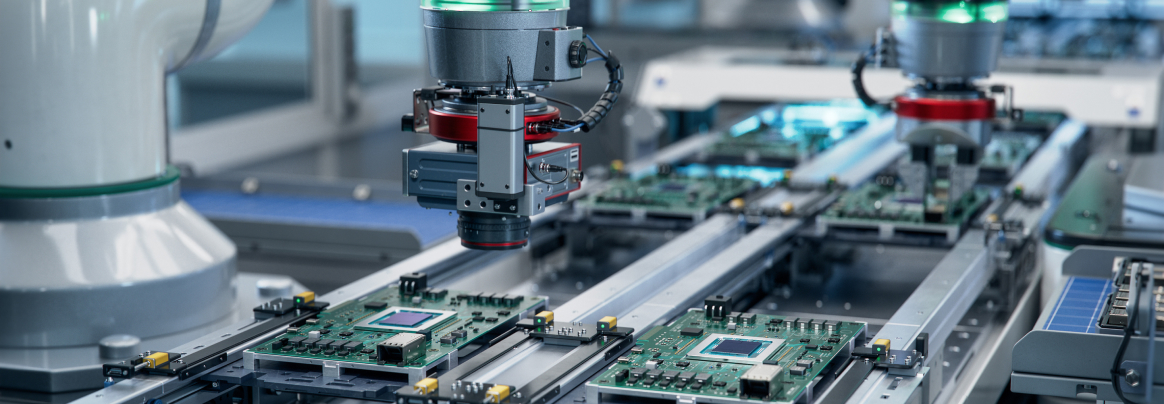
The solution
IoT + ML + data analytics for automated maintenance
Predictive maintenance (PdM) is a game-changer as it enables manufacturers to continuously monitor the condition of machinery and schedule service jobs only when necessary.
-
As manufacturers implement IoT sensors,
they get access to production data like temperature, vibration, energy consumption, etc. They can use this information to track the health of production machinery.
-
Using ML models and data analytics,
PdM tools analyse historical and real-time data to spot performance drops and enable timely intervention before issues escalate.
-
By integrating IoT with existing ERP and maintenance management systems,
manufacturers get a platform for tracking machine health and scheduling maintenance.
As a result, they can focus their efforts on where and when most needed to minimise downtime risks and optimise maintenance workflows.
Predictive maintenance in manufacturing: Results
The implementation of predictive maintenance delivers impressive results.
A01
First, it visibly reduces downtime
on average, between 30% and 50%—thanks to early detection of potential failures and monitoring of machinery health.
A02
Second, maintenance costs decrease by approximately 20-40%
thanks to optimised servicing schedules, increasing process automation, and reduced reliance on emergency repairs.
A03
A04
Finally, production schedules become more reliable,
boosting overall throughput and meeting customer demand on time.
Once the PdM pilot proves its value, manufacturers typically scale their predictive maintenance programmes to additional machinery to ensure consistency across facilities.
More robust AI algorithms help further optimise production flows by spotting new efficiencies, such as reducing energy consumption and boosting sustainability.