Challange
accurate risk assessment and pricing
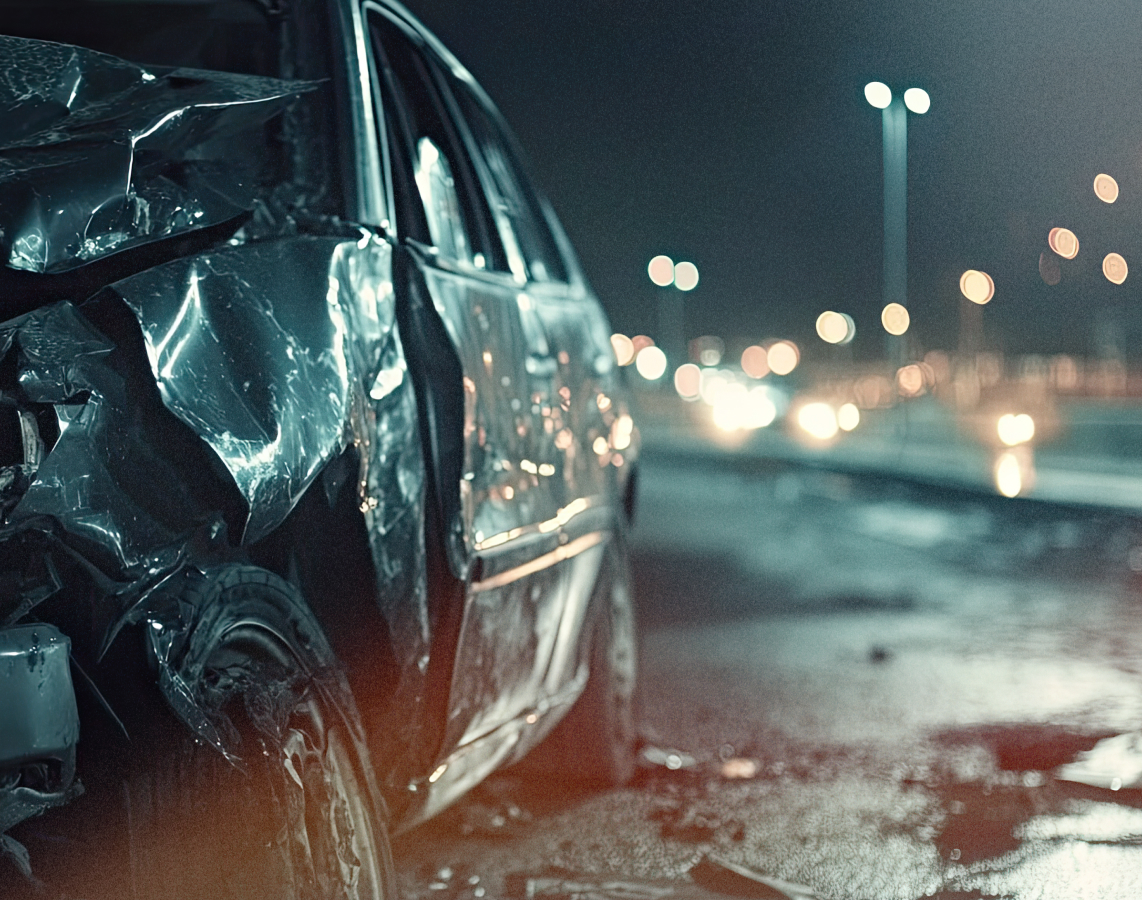
Precision in pricing risk has been a central issue for companies in the insurance industry.
Traditional risk models rely on static and historical data, understandably leaving room for inefficiencies. Real-world variables such as behavioural patterns and environmental conditions can add to the precision of pricing.
Yet, while real-time operational data is often accessible, insurers frequently face challenges incorporating it into their existing systems.
This results in broad-brush risk assessments that either underprice or overprice policies. A fast-evolving insurance market definitely calls for more precision if your company wants to stay competitive.
Assessing available options
When trying to improve insurance risk assessment, companies typically explore the following:
#1: Enhanced historical modelling
Using advanced actuarial techniques can provide incremental improvements, but they are still limited by the static, non-adaptive nature of historical data.
#2: Manual onsite insurance risk assessment
While effective in certain cases, these assessments are still too time—and resource-intensive and offer only a snapshot of conditions rather than consistent insights.
#3: Basic telematics solutions
Simple telematics can bring value to specific industries like automotive. However, these solutions still lack scalability and can’t fully address the needs of commercial insurers across all market segments.
While partly helpful, none of these solutions delivers the nuanced insights necessary for comprehensive risk assessment – and that’s where IoT has the potential to revolutionise the field.
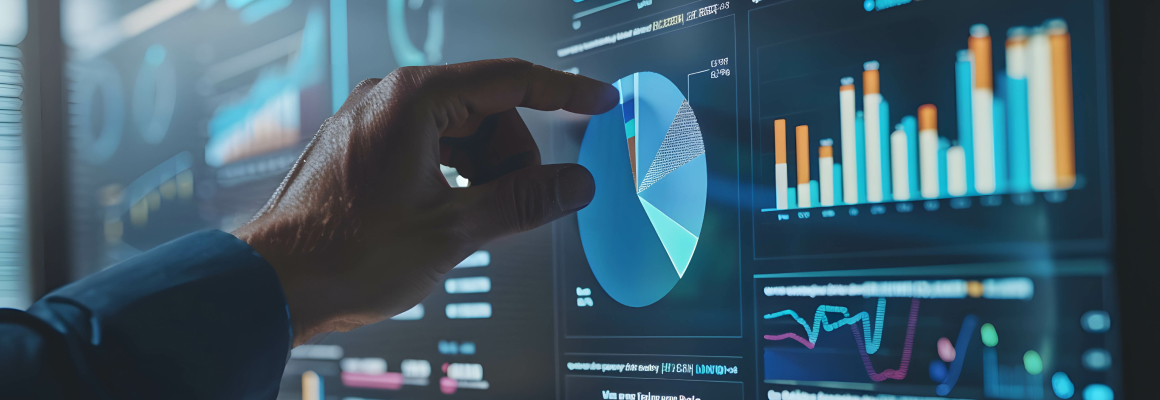
The best solution
IoT + ML + cloud computing + automation
-
Firstly, dynamic risk profiling.
Data streams continuously deliver insights into evolving risks, from machine wear-and-tear and health conditions to workplace safety conditions.
-
Secondly, expanded factors for pricing.
Insurers can shape pricing models with unparalleled precision thanks to variables like vibration metrics or usage patterns.
-
Thirdly, seamless integration with analytics platforms.
IoT solutions can send real-time data directly into underwriting platforms and Machine Learning tools for immediate analysis and decision-making.
Thanks to the cloud, the entire ecosystem can store and process large volumes of IoT data to ensure the required level of analysis with the built-in ML mechanisms.
Moreover, by automating parts of the process, insurers guarantee that their customers can enjoy much faster and more relevant service.
Results: more adequate insurance risk assessment
The adoption of IoT-driven insurance risk assessment brings astounding results:
A01
Firstly, improved pricing accuracy.
IoT-powered risk models are even 25% more precise than before, resulting in more competitive premiums.
A02
Secondly, enhanced customer satisfaction and retention
Thanks to more competitive policies, clients enjoy more affordable prices and incentives for maintaining low-risk profiles.
A03
Thirdly, better operational efficiency.
Automation and ML-driven insights significantly cut underwriting time.