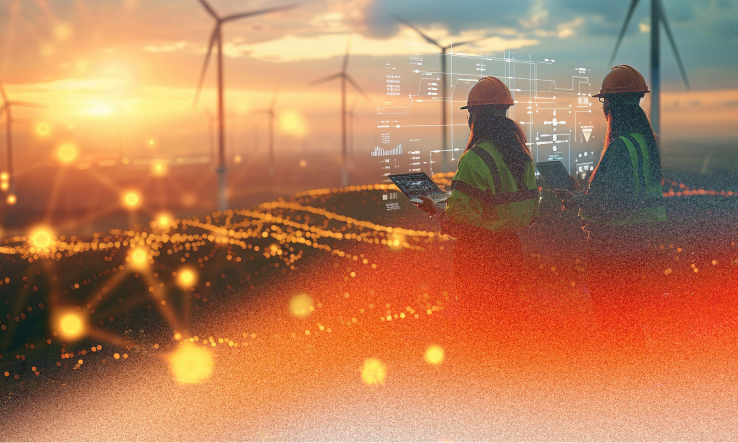
Manufacture
Unlocking the Potential of Predictive Maintenance for Your Production Business
Manufacturing downtime is costly, both in terms of finances and productivity.
According to research from Siemens, only an hour’s downtime in the automotive sector costs more than $2 million.
For an average manufacturing facility, downtime is an all-too-frequent occurrence.
On average, 20 unplanned monthly interruptions translate to a loss of about 25 production hours. You don’t need an advanced maths degree to realise the whopping costs of such halts.
These numbers highlight the significance of predictive maintenance (PdM) for production companies. Here’s what your manufacturing business needs to know about PdM to make the most of it.
What is predictive maintenance?
Predictive maintenance (PdM) maximises uptime by improving the reliability of production equipment and optimising its maintenance schedules.
As one of Industry 4.0’s cornerstones, predictive maintenance empowers businesses to move from reactive maintenance—fixing machinery after failure—to more proactive approaches. According to McKinsey, this shift can reduce equipment downtime by 30%–50% and increase machine life by 20%–40%.
By monitoring factors like sound, vibration, temperature, and oil lubrication levels, PdM solutions can spot anomalies and predict failures before they occur. Commonly monitored equipment elements include pumps, fans, compressors, motors, gearboxes, and bearings.
Benefits of predictive maintenance for manufacturers
Apart from the already mentioned reduction of downtime and related costs, PdM delivers the following benefits:
#1: Minimised repairs and optimised resources
Early issue detection reduces the need for extensive repairs and ensures optimal allocation of maintenance resources.
#2: Increased equipment lifespan
PdM minimises wear-and-tear by identifying and addressing issues early. This leads to improved Overall Equipment Efficiency (OEE), which often reaches 90%.
#3: Smooth operations
By preventing unexpected failures, PdM reduces production halts, ensuring uninterrupted operations.
#4: Enhanced safety
Preventing spectacular equipment failures also minimises the risk of workplace accidents, protecting your employees and facilities.
How predictive maintenance works
Predictive maintenance runs on data and uses advanced technologies to predict and prevent equipment failures.
Here’s a look at the key enablers of PdM:
- Internet of Things (IoT): Smart sensors collect real-time data on equipment performance, including temperature, vibration, and pressure.
- Cloud computing provides centralised data storage and access, ensuring all stakeholders can retrieve insights anytime, anywhere.
- Artificial Intelligence processes vast datasets to uncover patterns, establish baseline, and spot anomalies.
- Machine Learning models predict potential failures by analysing historical and real-time data and capturing the relationship between equipment performance metrics and degradation.
By combining these technologies, PdM solutions can estimate the occurrence of potential failures, enabling you to schedule maintenance tasks at the most efficient intervals.
Now, consider a few examples to understand better how predictive maintenance can empower your production processes.
Real-life examples of PdM
Predictive maintenance in FMCG:
Frito-Lay is a US subsidiary of PepsiCo, producing crisps and multiple snack foods.
The company implemented predictive maintenance tools across its most critical assets in the Tennessee plant. The system remotely monitors vibrations, analyses the data, and notifies the operator in case of any irregularities; it also uses infrared analysis on electrical equipment, heat exchangers, and additional components.
Vibration readings confirmed by ultrasound allowed the company to prevent a failure of a PC combustion blower motor, which would shut down the whole potato chip department.
Moreover, the producer detected oil degradation by spotting higher acid levels in oil samples from a baked extruder gearbox and avoided shutting down its entire production unit.
Predictive maintenance in energy:
Over 60% of San Diego Gas & Electric (SDG&E) assets are underground and approaching 40+ years of age. The company uses the potential of AI, ML, and Computer Vision to improve its maintenance and risk management.
One of its initiatives uses ML algorithms to accurately predict and prevent T-splice failures. The solution correlates high-frequency sub-cycle data with other system data, letting algorithms recognise anomalies and signs of asset failure in near real-time.
Another project uses AI/ML and Computer Vision to automatically spot assets and damages on the utility’s electric system for better maintenance plans and wildfire risk reduction. Drones capture images of equipment, while ML models spot damaged assets and prioritise them for repairs.
Predictive maintenance challenges
Although PdM offers impressive benefits, it’s not suitable for every production company—at least not immediately.
Implementing predictive maintenance requires thoughtful planning and investment. Here are the most common challenges to consider beforehand:
#1: Your current infrastructure
PdM often requires upgrading existing systems and integrating advanced monitoring tools. Assessing your current infrastructure and determining if additional investments are necessary in your setup is essential.
#2: Internal knowledge & staff training
Your team must have the know-how and get guidance on operating PdM systems. Building awareness of the benefits and providing hands-on training are necessary steps to unlocking your business’s potential for predictive maintenance.
#3: Data collection capabilities
PdM relies on large volumes of data for accurate predictions. If your organisation doesn’t collect and process relevant production data, you must establish data pipelines before implementing PdM.
Your organisation must already gather and process data adequately, or you risk losing time and delaying the ROI of your PdM solution.
Six steps to implement predictive maintenance
Here’s a high-level roadmap for production companies wishing to adopt PdM:
#1: Assess your PdM readiness
Evaluate your current systems, data collection capabilities, and workforce skills. Identify gaps and prioritise necessary upgrades.
#2: Choose the right technologies and tools
Select IoT sensors and data platforms that align with your operational needs. Ensure compatibility with your existing infrastructure and IT systems.
#3: Build a data strategy
Establish a robust data pipeline that collects, stores, and processes your production equipment performance data.
#4: Develop AI and ML models
Develop AI and ML models tailored to your equipment and processes by working with your in-house team or enlisting external AI and data experts.
#5: Train your team
Ensure your team gets comprehensive training and can effectively use, maintain, and develop PdM systems.
#6: Pilot and scale
Start small with a pilot program on your most critical equipment, then scale PdM across your facility once you validate its effectiveness.
Conclusion
Predictive maintenance is a transformative strategy for modern manufacturers. By addressing challenges like unplanned downtime, high repair costs, and safety risks, PdM has the potential to become a game-changer in the production industry.
By investing in PdM, businesses enhance operational efficiency, extend equipment lifespans, and maintain a competitive edge. However, predictive maintenance may require significant financial investment, time, and preparation before your company can fully reap its benefits.
Ready to explore how predictive maintenance can boost your manufacturing processes? Contact us today for a free consultation on your PdM readiness assessment.